The Quiet Risk in AI Nobody Talks About
When people talk about AI risks, they usually focus on the model itself: the algorithm, the output, the black box logic. But there’s a quieter, earlier issue that often gets overlooked. One that starts before the model is even trained. Mislabeling.
AI models rely on labeled data to learn. If that data is wrong, the model learns the wrong patterns. It’s that simple. And while it sounds like a minor issue, the impact can be huge. You don’t need thousands of bad labels to tank performance. Sometimes, just a few flawed examples can shift the behavior of a system meant to serve millions.
Most teams assume bias comes from the model or the math. But in reality, it often starts with a human decision. Someone interpreting a sentence. Tagging an image. Judging intent in a snippet of audio. If those decisions are rushed or unclear, the training data starts to drift. And once that happens, your model isn’t just inaccurate. It’s confidently wrong.
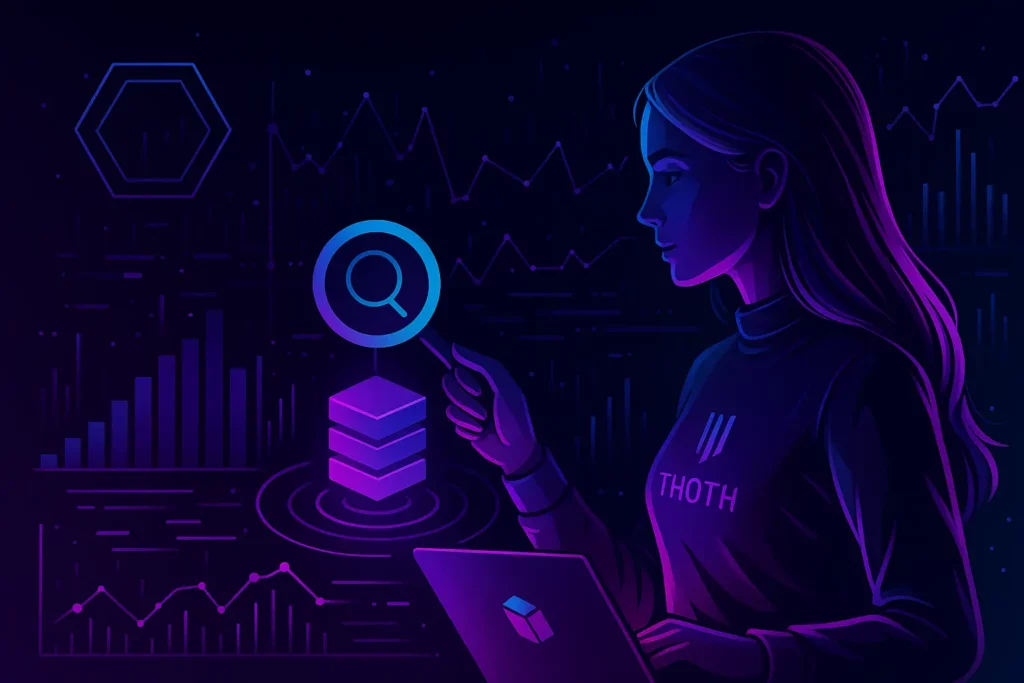
Why Labeling Goes Wrong
Labeling sounds straightforward. A task gets assigned. A label is chosen. Done. But in practice, there are many ways for it to break down.
Sometimes the input itself is ambiguous. A blurry photo, a sarcastic tweet, a sentence out of context. Other times, two annotators interpret the same example differently. Especially when guidelines are vague or evolving. And often, it comes down to pressure. Teams are racing to label millions of items without enough time to review or refine.
Once those labels are locked in and used for training, the system begins to reflect those assumptions. This doesn’t just lead to slightly worse predictions. It can create serious problems.
What Mislabeling Actually Affects
Mislabeling can cause models to make errors in unexpected ways. Some of the common effects include:
- Misclassifying sensitive categories like gender or emotion
- Reinforcing cultural or language biases
- Ignoring less common but important edge cases
- Producing outputs that seem confident but are fundamentally off-base
These issues often go unnoticed in testing. However, once the model is deployed, it can impact user experience, lead to exclusion, or even introduce legal and ethical concerns. And by the time teams notice, the problem has already been baked in.
How to Catch It Before It Spreads
Fixing these issues isn’t about adding more compute or tuning hyperparameters. It starts earlier. At Thoth AI, we take a different approach. Our goal is to make sure the data your system learns from is as reliable as possible.
That includes:
- Creating detailed guidelines for every labeling task, with examples and edge cases included
- Running multiple review rounds to catch inconsistencies
- Using escalation paths for unclear examples rather than forcing a quick decision
- Running regular audits on labeled data to check for bias, drift, or blind spots
We also revisit old datasets when performance dips or user complaints spike. Models evolve, but so do the patterns in user behavior and the data itself. A dataset that worked two years ago might be leading your model astray today.
Why This Matters for Businesses
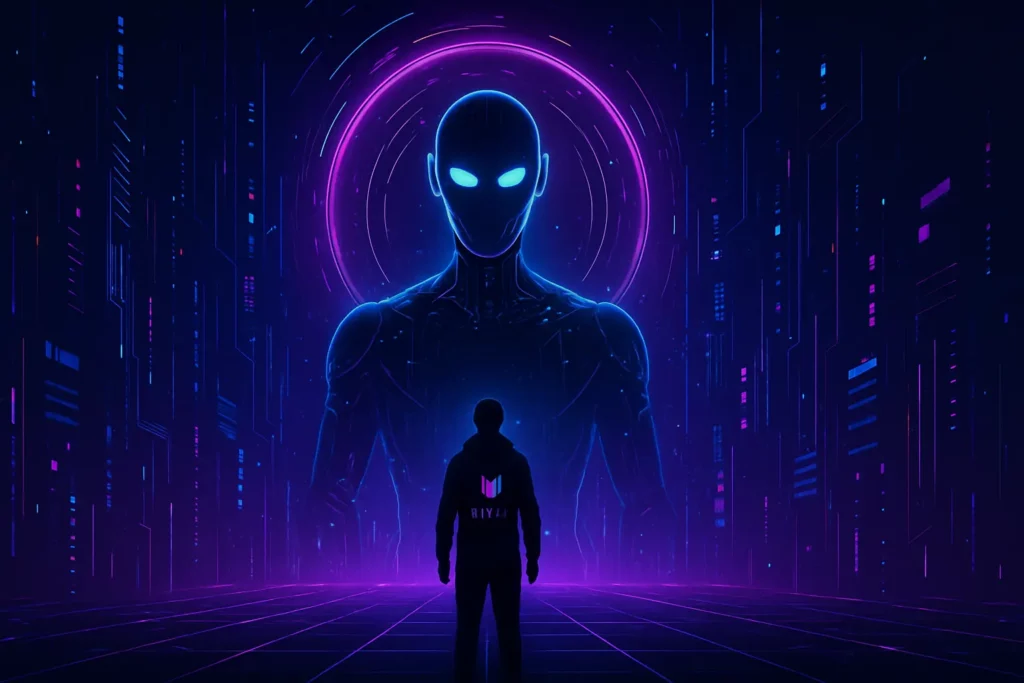
Good labels don’t just improve model accuracy. They reduce risk. They make your system more adaptable to changing environments. And most importantly, they make it easier to trust the outputs your model is producing.
That trust matters. Whether your AI is making recommendations, screening content, or supporting decision-making, users expect a certain level of reliability.
If something feels off, it undermines the entire system. On the flip side, when predictions feel aligned with real-world experience, confidence grows.
Investing in proper labeling workflows isn’t just a technical fix. It’s a governance choice. One that shows your organization is serious about building AI that’s not only effective but also responsible.
It’s Time to Treat Labeling as Core Infrastructure
Many companies still treat data labeling as a side task. Something outsourced or rushed through. But the reality is, it’s foundational. If your model is built on shaky ground, no amount of fine-tuning can truly fix it.
Think of labeling as quality control for your AI. You wouldn’t let flawed ingredients into a manufacturing line. The same logic should apply to your training data.
If you want an AI system that performs well in the real world, it has to be trained on data that reflects it. That means clear standards, careful review, and a willingness to recheck assumptions as your system grows.
At Thoth AI, we help companies build and maintain that standard. Because great AI doesn’t start with algorithms, it starts with better data.